How Edge AI is Redefining Data-driven Decision Making in Industrial Automation
Integration of AI, cloud computing, and edge technologies is driving a new wave of innovation in industrial automation. While most traditional IoT solutions still rely on basic telemetry, collecting data from edge devices and storing it centrally, modern approaches are moving well beyond this. By bringing intelligence directly to the edge, Edge AI enables real-time decision-making, reduces latency, and transforms how machines operate on the factory floor.
The use of state-of-the-art machine-learning and deep-learning models as Random Forest, K-Means, DBSCAN, and Convolution Neural Networks, YOLO, and others to address real-world problems in industries such as quality control, collecting relevant data, informed decision making, compliance, predictive maintenance, and asset tracking. These models can be trained for the task of practicing on the cloud, and the solutions can be directly applied to the edge device.
Edge AI optimizes business processes via feedback loops as you let real-time decision making with the use of AI deployments into the edge with TinyML, YOLOv5, and many others. By enabling localized intelligence, it can create continuous feedback loops that optimize operations and enable real time decision-making.
As per recent analysis by KBV Research, a rapid adoption of intelligent edge technologies across industries and estimated to drive the global market for edge AI in Industrial Automation will reach $268.5 billion by 2031, rising at a CAGR of 25.4%.
Why Traditional Cloud-Dependent Models Fall Short
Traditional cloud-dependent systems, however, face several challenges:
- Latency Issues: In time-critical processes, even minor delays can lead to serious consequences. For instance, in robotics or automation, a delay in receiving sensor data could hamper a system, such as a robot, which could perform inappropriately, leading to a hazard or accident.
- Bandwidth and Network Reliability: Industrial Internet of Things (IIoT) devices generate large streams of data, which may affect the network infrastructure. The outcome of these effects causes network overload and high latency. Bandwidth limitations can also limit the amount of data that can be transferred at any given time.
- Privacy and Security Concerns: Critical data information, including measurements of manufacturing, control systems, and intellectual property, which are common parts of industrial processes. If this data is not well structured and secure with proper safeguard measures, industries can face significant security hazards if any such critical data is sent to the cloud. Whereas strong security protocols such as access control, two factor authentication, and encryption are necessary to prevent unauthenticated access and breaches.
- Scalability and Device Management Complexity: Cloud-based management of a substantial fleet of interconnected edge devices brings an immense amount of complexity, particularly as implementations expand across various locations.
What Edge AI Brings to Industrial Automation
Edge AI simplifies the process of industries with on-device deployment of AI, with essential corrections that are required for upgrading the operations of industries. It is the driving force of recent industries’ development and transformation of existing industries by faster, secure, and cost-effective solutions, where industries can leverage more time-saving, faster production, and mitigate errors in production lines that enhance production efficiency, and real-time analytics.
- Immediate Data Processing
One of the most remarkable benefits of Edge AI is its potential to process data at the point of generation. Some significant volumes of operational data, including temperature, vibration, pressure, speed, and many more, are generated by sensors and machinery in industrial facilities. Edge AI also shares benefits with real-time analysis of the data on the device without shifting it to the cloud. As a result, systems are efficient in responding promptly to major changes, including detecting malfunctions in machinery or precisely tweaking the movement of the robotic arm in milliseconds.
- Reduced Data Transfer
The traditional systems often share a vast amount of raw data to the cloud, where every kind of analysis of various data can be done using a higher amount of bandwidth, and this increases significant operational expenses. Edge AI reduces this load by analysing and sorting the data locally and delivering only the most important and filtered insights to the cloud. For instance, an edge-enabled sensor can identify bugs or anomalies and only transmit exceptions, instead of streaming constant data. In addition to sparing network resources, this minimizes data transfer and assures more seamless functioning in limited bandwidth.
- Enhanced System Resilience
Industrial processes and aligned work cannot tolerate a single interruption between the processes due to uncertain connectivity. Edge AI evolves a sensitive layer of durability that ensures that decision-making and automation can be uninterrupted even in the loss of internet connection. Devices and machinery can still be in operation and reliably perform tasks, analyse data, and easily navigate their actions locally without relying on cloud access. This autonomy improves uptime, safety, and process continuity in any environment where network continuity cannot be there.
Edge AI Use Cases in Industrial Environments
1. Predictive Maintenance
Edge AI can be used to monitor real-time measures of temperature, vibration and pressure with the use of IoT sensors. By evaluating this data locally, AI models can identify wear or malfunction critical systems early and predict challenges before they occur. This reduces maintenance costs, increases equipment lifespan, and decreases uncertain downtime. Edge AI can assist in taking prompt decisions in any such harsh situations, in contrast to cloud-based monitoring.
- Compact AI Models: Compact AI Models: Techniques like quantization and model compression, which can operate predictive maintenance algorithms effectively on low-power edge devices without diminishing accuracy
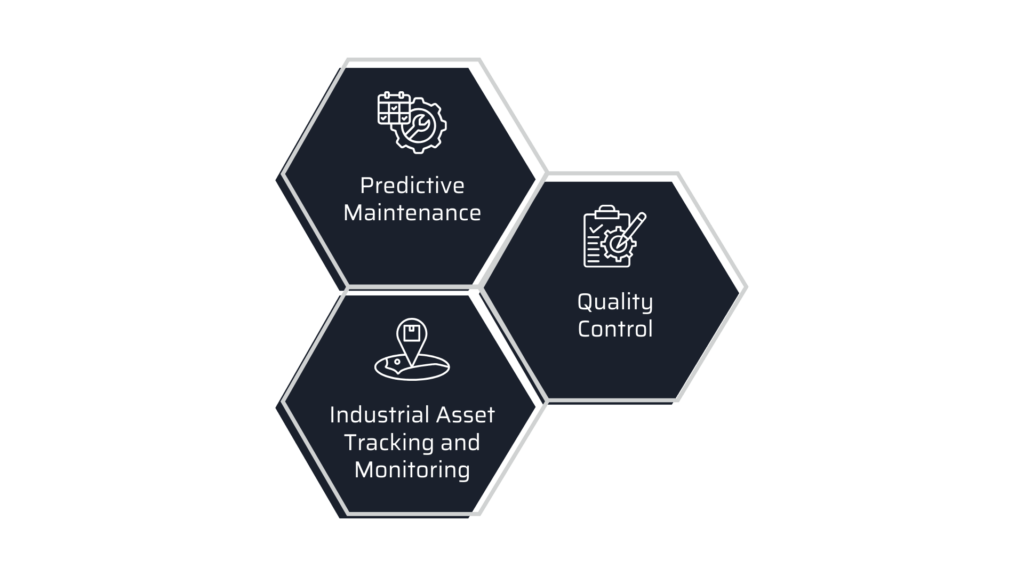
Edge AI Use Cases in Industrial Environments
2. Quality Control
Manufacturers can inspect product quality on an assembly line within milliseconds with the help of Edge AI-powered computer vision systems. AI models can be ported on cameras or embedded devices swiftly to detect flaws, assuring the products which is having quality assurance marks that has to be proceed further. This reduces dependence on manual inspection, enhances consistency, and lowers the material waste.
- Low Power AI Hardware: Real-time vision models can run actively on the edge with the support of platforms like MPSoCs and FPGAs, which enables high-speed range image processing in industries with minimum power consumption.
3. Industrial Asset Tracking and Monitoring
Industrial Asset Tracking in industrial workspace entails real-time monitoring tools, machines, and inventory to promote effective functions. Edge AI processes data from RFID, UWB, or vision systems locally, minimizing latency and allowing real-time updates without relying upon cloud processing. This improves logistics, reduces asset loss, and boosts operational visibility.
- Real Time Operating Systems (RTOS): Adoption of Real Time Operating Systems (RTOS) such as Zephyr or FreeRTOS, which provides predictable task scheduling for efficient data processing management. Furthermore, sensor fusion and protocol support ensure smooth communication and incorporation with outdated industrial machinery.
All in all, Edge AI plays a key role in enabling critical decision-making. In the future, we can expect more computational power coming to the edge to assist industries in making decisions faster and reduce the chances of production breakdowns. The emerging AI models in the future could be more effective with a higher accuracy rate, lighter, and real-time inference. Industries will enjoy autonomy, reduced latency, and a lot of resilience with more AI-powered edge devices, such as MPSoCs, FPGAs, and NPUs. The processes will be more flexible in facing changes shortly because they will integrate with Free Real-Time Operating Systems (RTOS) like Zephyr, FreeRTOS, or any other emerging OS.
With 25 years of experience across Silicon and Product Engineering, MosChip has developed and delivered over 500 products and created over 75 AI models ready to be deployed across various platforms. We our proven expertise, and solutions Ips and accelerators our service offerings are poised to accelerate product development and time to market.
Leveraging this expertise, MosChip enables industrial automation with Edge AI solutions, using low-power, high-performance hardware such as MPSoCs, FPGAs, and NPUs. Additionally, it supports real-time operating systems (RTOS) like Zephyr and FreeRTOS for consistent task completion and offers seamless compatibility with existing systems through advanced sensor fusion and protocol support. To know more about MosChip’s capabilities, drop us a line and our team will get back to you.
Author
Darshil is a Marketing professional at MosChip creating impactful techno-commercial writeups and conducting extensive market research to promote businesses on various platforms. He has been a passionate marketer for more than four years and is constantly looking for new endeavors to take on. When He’s not working, Darshil can be found reading and playing guitar.